Rockstar technology has world of possibilities
11 August 2022
The oldest human ‘technology’, stone artefacts, can now be distinguished from rock by the most sophisticated of technologies; artificial intelligence.
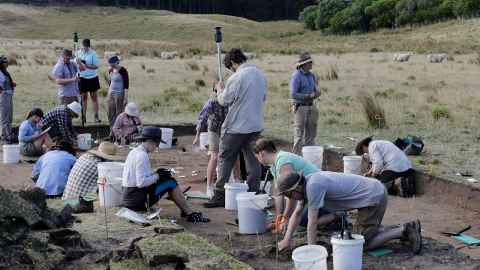
A University of Auckland archaeology team, led by postdoctoral fellow Dr Joshua Emmitt, trained a computer algorithm to distinguish stone artefacts from natural rocks with 100 percent accuracy.
The model outperformed two human experts who examined a sample of the same images used to train the machine, and has meant thousands of images taken in the field in Egypt, Australia and Aotearoa over the past 20 years have now been quickly and efficiently categorised.
Dr Emmitt says this process, which is now being built on and expanded by a transdisciplinary team at the University, has exciting implications for archaeological research and human knowledge.
“Archaeologists often only examine a fraction of what is identified during fieldwork due to time constraints, so the ability to rapidly identify material means that entire assemblages (a group of different artefacts found close to each other) can be examined in this way.”
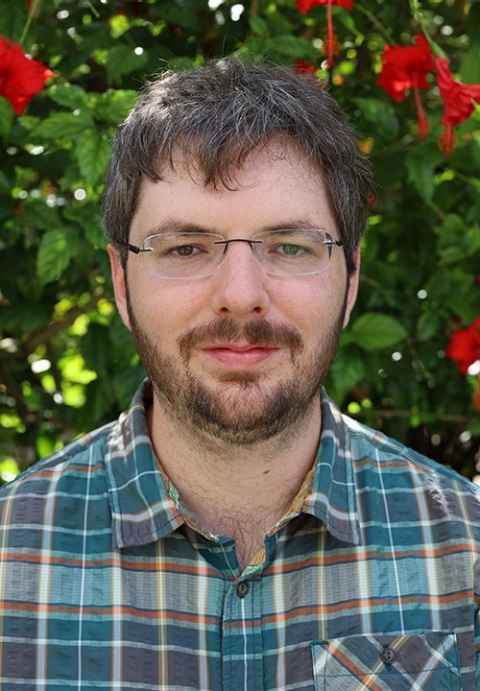
He says this sort of AI technology was originally developed over the last decade to enable driverless cars to tell the difference between approaching cars, humans and other objects, but has only more recently been used in the field of archaeology.
And while his team are not the only ones using it worldwide, they are the first to apply it to this particular set of images with this criteria.
“I was thinking about this possibility and working with the University’s Centre for eResearch (Faculty of Science) as far back as 2012, but it’s just taken time for the algorithms to become more efficient and accessible to us so we could apply them.”
He says training a machine to do a job that has a noted shortage of skilled practitioners also frees up valuable human resources for other work computers can’t do.
And although people might think fully formed tools are the only things of interest to archaeologists, Dr Emmitt says in fact they make up only around one percent of what there is to discover in any given survey area; hence the value of the machine.
This single determination between rock and artefact, from a two-dimensional image, was our primary goal with this research, but of course we’re hoping it can be the basis for many more studies.
“Being able to quickly identify large numbers of ‘cores’ and ‘flakes’ for example – stone flaking involves striking pieces (flake) off a larger rock (core) – across a particular area gives archaeologists a much better idea of how humans moved through the landscape and how the sites were originally formed.”
He says this is a first step for the algorithm, which could eventually be programmed to recognise more complex types of artefacts.
“This single determination between rock and artefact, from a two-dimensional image, was our primary goal with this research, but of course we’re hoping it can be the basis for many more studies.”
Dr Emmitt’s research was done in collaboration with Dr Sina Masoud-Ansari, formerly from the Centre for eResearch, Dr Rebecca Phillipps, a senior lecturer in anthropology, research assistant Stacey Middleton, Jennifer Graydon, a summer scholar in anthropology and Professor Simon Holdaway, Associate Deputy Vice-Chancellor (Research) at the University of Auckland.
While it uses already existing data funded from many sources, the current research was started as a summer scholar’s scoping project and developed as part of ongoing research. Jennifer Graydon’s involvement was made possible by a Natalie Blair Memorial Summer Scholarship in Archaeology.
Machine learning for stone artifact identification: Distinguishing worked stone artifacts from natural clasts using deep neural networks by Joshua Emmitt, Sina Masoud-Ansari, Rebecca Phillipps, Stacey Middleton, Jennifer Graydon and Simon Holdaway has been published in PLOS One.
Media contact
Julianne Evans | Media adviser
M: 027 562 5868
E: julianne.evans@auckland.ac.nz