Masters research project/dissertation
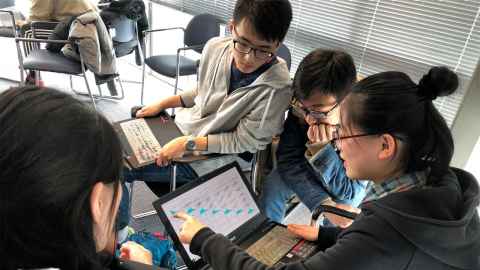
All of our Master of Professional Studies (Data Science) and Master of Data Science students are required to undertake a research project/dissertation worth either 30 or 45 points. These projects are conducted either part-time, across the university year, or full-time during one semester.
To meet the requirements of their research project/dissertation, students will either complete an academic research project with academic supervisors, or an industry project guided by a team of academic supervisors and industry mentors, giving students experience solving real-world problems. Projects may be undertaken individually or in groups (where appropriate).
Project ideas come from industry partners – they could be looking for a creative solution to a problem, or perhaps have a nice-to-do project that they simply haven’t had the resources to get underway. In return, industry partners can benefit from innovative and cutting-edge solutions, and perhaps use the opportunity to identify students for future job opportunities.
What is the difference between a project and an internship?
Industry projects differ from internships in that students are not necessarily situated at the industry partner’s place of business for the project duration. Students are predominantly located at the University, however there may be instances where they are required to be located at the company (for example, to access data, or to meet with their mentor/project team) and we find that when students are introduced to the wider business and its operations they get more value out of the experience. Students will have an academic supervisor based at the University, and an industry mentor or project contact to assist with the project.
Projects require a clear scope, discussed between the industry partner, the academic supervisor and the student. Students are expected to produce a research project/dissertation that demonstrates a capacity for independent thinking, contributes to existing scholarship, and meets the standards for scholarly research.
If the project is for a full-time masters student completing it within one semester, the project should be well-defined and the data needs to be available at the start of the project.
If the project is discovery-based, it may be more suitable for a part-time student, completing it over two semesters. Most of the resources should be available at the start of the project, but there is more scope to add resources (such as new data) during the project.
Typical project areas
- Predictive modelling and developing forecasts, for example, customer churn, the seasonal demand for products or the performance of certain markets.
- The application of machine learning and data mining to a particular domain, such as classification tasks, deep learning or the discovery of patterns in data.
- Data, information and knowledge management, such as the introduction of new technology, data cleaning tasks, information integration and knowledge representation and retention.
Benefits for industry partners
- Complete advanced projects for minimal investment
- Mentoring opportunities to steer the project to meet your business needs
- Get in-depth and cutting-edge research for your company
- Exercise your corporate citizenship and participate in education
- Establish connections with the University for future collaboration
- Identify top students for recruitment
- Intellectual property is negotiable
- Confidentiality is ensured – there is the possibility to embargo a research project/dissertation if the results are sensitive or significant
Role of the industry partner
- Provides mentor/project contact/subject matter expert for 1-3 hours per week
- Gives access to any specialist equipment required
- Provides IT support, software, and other resources needed to perform successfully in the project
- Ensures a positive and supportive management framework
How it works
- Industry partners submit an expression of interest and project description, usually by 31 January for Semester One start, and 31 May for Semester Two start, of the relevant year.
- The University of Auckland will provide a selection of students who have expressed interest in the projects, and will facilitate interviews between students and industry partners.
- The industry partner selects the student, and the University assigns an academic supervisor.
- Students complete their research project/dissertation over either a semester or a university year (two semesters), with a report and final presentation upon completion. The University will host a research showcase at which the students will present their work.
The students
Students in the Master of Professional Studies (Data Science) will generally have a background in a data science-related area and at least three years’ relevant work experience. As part of their studies they will undertake courses in Advanced Database Systems, Big Data Management, Advanced Machine Learning, Statistical Datamining, and Statistical Computing.
Students in the Master of Data Science will have completed an undergraduate degree with a major in Data Science or a double major in Statistics and Computer Science, and will undertake courses in Big Data Management, Datamining and Machine Learning, Advanced Regression Methodology and Advanced Data Science Practice.
A student completing their 30-point research project part-time will commit 10 hours/week to the project for around 28 weeks. The student will complete the work over two semesters and is required to complete 300 hours of work.
A student completing their 30-point research project full-time will commit 20 hours/week to the project for 14 weeks (one semester) and is required to complete 300 hours of work.
A student completing their 45-point dissertation part-time will commit 10 hours/week to the project in the first semester and 20 hours/week in the second semester, and is required to complete 450 hours of work.
A student completing their 45-point dissertation full-time will commit 30 hours/week to the project for 14 weeks, and is required to complete 450 hours of work.
Academic supervisors
Students will be supervised by an academic (or academics) from within the University, usually from the School of Computer Science and/or the Department of Statistics, but where appropriate, supervisors may come from the Faculties of Business and Engineering.
Project examples
- Business Register Extended Coverage (Statistics NZ), Venu Nair, 2016
- Applying Big Data Technology on Mobile Datasets (Datamine, Ltd.), Eric Jin, 2016
- Weather-based Insights and Other Factors on Museum Visitations (Dexibit), Norman Yap, 2017
- Sentiment Analysis with Email Data for Sales Prediction (Callaghan Innovation), Punit Kumar Rajgarhia, 2017
- Predicting Imminent Changes of Fair Value for Nikkei 225 Futures Index on Basis of Order Book Data (TRV Trading), Neil Moraes, 2018
- Forecast of Skim Milk Powder Exports for Fonterra (Fonterra NZ), Jason Tam, 2018
- A Data Visualisation Application for Rugby Coaching using R Shiny (Auckland Rugby), Yi Dai, 2019
- Automation of Product Mappings Across Different Datasets (VESTA), Jackie Excell, 2019
Industry partner feedback
“Yi Dai (Master of Professional Studies (Data Science), 2019) teamed up with Auckland Rugby Union for his dissertation project. Yi applied advanced tools from AI, machine learning and statistics to training and game data. He designed and implemented a data visualisation app that makes it easier to understand rugby data, the performance of players and the correlation between training units and key performance indicators in games. Yi’s tool will inform decisions about the training of players and game strategy at Auckland Rugby on a continued basis.”
Paul Downes, Athletic Performance Director, Auckland Rugby Union
“All three projects the Vesta team have undertaken with Data Science students have brought considerable R&D value to the business, especially in being able to research global best practices and methods of emerging data science and digital technologies outside of the live Vesta platform environment. The students were of exceptionally high calibre both in terms of maturity and responsibility, plus intellectually and technically competent. This made managing and supervising projects very easy. We highly recommend these types of projects, especially with Callaghan R&D support, and engaging with staff and students at the University of Auckland was easy and professional.”
Tim Harris, Vesta eCommerce
Student testimonials
"Thanks to the recommendations of Prof Sebastian Link and Kylie Chye, I have an opportunity to do an industry project with Watercare. The whole process of working on the project gives me more practical experience and helps me to make rapid progress. Watercare sent professional engineers to explain the project requirements and supply the data set and my high-level supervisor gives me great guidance on my project. I can apply my knowledge to practice to solve problems. This industry project opportunity is, for me, the best part of studying at the University."
Wei Xie, Student, MProfStuds (Data Science), 2019
"All the learning during the course and right selections with your help all the way through including the industry project with Callaghan Innovation did make a huge difference in interviews. Thanks a ton for all your guidance and help!!"
Punit Kumar Rajgarhia, Graduate, MProfStuds (Data Science), 2017
“The MProfStuds (Data Science) programme offered the ideal combination of taught papers and research to compliment my existing training in Public Health. I was fortunate enough to become involved with a research team who had developed a self-administered psychosocial screening tool for young people called YouthCHAT used in GP Clinics and schools.
Leveraging the techniques taught in the first semester I developed a prototype analytics portal to facilitate analysis of the amassed collection of de-identified data. The benefits of the research included equipping users, say the practice manager, with the ability to monitor trends in their data and to assist in resource allocation planning. For example, if one type of psychosocial issue was more pertinent within a clinic, a specialist may be recruited. I benefited by being exposed to the process of collaborative refinement with stakeholders, building expertise in R, human computer interaction, and becoming proficient in constructing interactive tools with visualization components.
I use these proficiencies often in my current position, and have built similar tools, including those to help researchers browse metadata on longitudinal studies and to facilitate a level of self-service with less user-friendly data formats.”
Ben Elliott, Graduate, MProfStuds (Data Science), 2018.
Connect with us
Need more information?
To register your interest, or if you would like further information about industry projects, contact our Industry Liaison Coordinator at datascienceprojects@auckland.ac.nz.