Astrostatistics research
At the intersection of observational astronomy, statistics, and data science, astrostatistics focuses on statistical problems in astronomy and astrophysics.
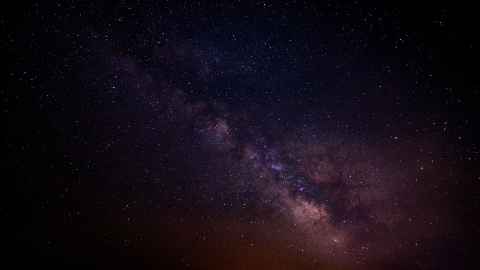
Large data challenges
Astronomical data poses large data analysis challenges across many branches of statistics such as signal processing and time series, image and spatial analysis, multivariate, survival and extreme value analysis, and machine learning. Astrostatistics offers an attractive area of study for statisticians who are interested in complex and challenging problems.
International collaborations
We work on gravitational lensing and source detection, and methods for the analysis of astronomical time series data, with a special focus on gravitational wave data. We have established an international network of cross-disciplinary collaborations.
Related research organisations
NZ Astrostatistics and General Relativity Working Group
Laser Interferometer Gravitational-Wave Observatory (LIGO)
Laser Interferometer Space Antenna (LISA)
Our researchers
Dr Brendon Brewer
- Bayesian astrostatistics
- Bayesian inference, information theory, and related topics
- Computational methods (particularly nested sampling)
Dr Kate Lee
- Machine learning
- Approximate Bayesian methods
- Inverse problems
- LISA data analysis
Professor Renate Meyer
- Bayesian nonparametric methods
- Markov chain Monte Carlo methods
- Parameter estimation for gravitational-wave data
- Bayesian spectral density estimation
- LISA data analysis
Dr Patricio Maturana Russel
- Bayesian spectral density estimation
- Locally stationary time series
- Marginal likelihood, nested sampling
- LISA data analysis
Dr Matthew Edwards
- Bayesian nonparametric methods
- Bayesian spectral density estimation
- Gravitational wave data analysis for LIGO and LISA
- Deep learning